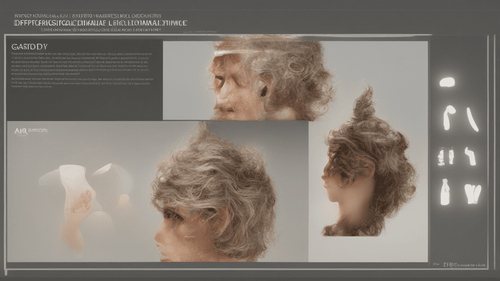
Introduction
In the rapidly evolving world of artificial intelligence (AI) and machine learning, Explainable AI has emerged as a critical component to unlock the black box of complex algorithms. As AI applications become more widespread, the need for understanding and interpreting the decisions made by these systems grows more important. In this comprehensive article, we delve deep into the concept of Explainable AI, exploring its significance, techniques, and benefits for businesses and society. Join us on this journey as we demystify the wonders of Explainable AI and how it is transforming the landscape of machine learning.
Understanding Explainable AI
Explainable AI, often abbreviated as XAI, refers to the methods and techniques employed to make AI models more transparent and interpretable. Traditional machine learning models, such as deep neural networks, often operate as black boxes, making it challenging to understand the reasoning behind their predictions or decisions. Explainable AI aims to shed light on these "black boxes," providing insights into the inner workings of AI models and the factors influencing their outputs.
The Significance of Explainable AI
Explainable AI plays a pivotal role in building trust and reliability in AI systems. By understanding the reasons behind AI model outputs, businesses, regulators, and users can gain confidence in the technology's decision-making process. Additionally, Explainable AI is essential for ensuring ethical AI practices and compliance with legal requirements, especially in critical domains such as healthcare, finance, and autonomous vehicles.
Techniques for Explainable AI
There are several techniques employed in the realm of Explainable AI. Some popular methods include:
1. LIME (Local Interpretable Model-agnostic Explanations)
LIME is a model-agnostic method that provides explanations for individual predictions. It generates interpretable models around specific instances to understand how the AI model arrives at its decisions for those instances.
2. SHAP (SHapley Additive exPlanations)
SHAP is based on cooperative game theory and assigns each feature's importance to the final prediction. It offers a holistic view of feature contributions and their impact on the model's output.
3. Grad-CAM (Gradient-weighted Class Activation Mapping)
Grad-CAM provides visual explanations for convolutional neural networks, highlighting the regions in an input image that the model considers significant for its decision.
Benefits of Explainable AI
The adoption of Explainable AI yields several advantages:
Enhanced Model Trustworthiness
By making AI models more transparent, Explainable AI instills trust and confidence in users, allowing for widespread acceptance and adoption.
Insights into Model Biases
Explainable AI helps identify biases and discriminatory patterns present in AI models, allowing organizations to rectify and mitigate potential harmful consequences.
Compliance and Regulatory Adherence
Industries governed by strict regulations, such as healthcare and finance, can utilize Explainable AI to comply with transparency and interpretability requirements.
Improved Decision-Making
By understanding AI model reasoning, stakeholders can make more informed and accurate decisions, leading to improved outcomes.
Explainable AI Techniques in Action
Let's explore some real-life use cases where Explainable AI has proven its value:
1. Healthcare Diagnostics and Treatment
Explainable AI has revolutionized healthcare by providing clear insights into medical diagnoses and treatment plans. Medical professionals can now understand why an AI system recommends a particular treatment, boosting patient trust and enabling personalized healthcare.
2. Financial Fraud Detection
Banks and financial institutions rely on Explainable AI to detect fraudulent activities. The system can explain why a particular transaction is flagged as suspicious, assisting investigators in making informed decisions.
3. Autonomous Vehicles
Explainable AI is crucial for the widespread adoption of autonomous vehicles. By understanding the reasoning behind an autonomous car's actions, passengers and pedestrians can trust the technology for safe and responsible driving.
4. Credit Risk Assessment
In the finance industry, Explainable AI helps assess an individual's credit risk by revealing the key factors influencing the creditworthiness of applicants.
Addressing the Challenges of Explainable AI
While Explainable AI offers numerous benefits, it also comes with certain challenges:
1. Trade-off between Complexity and Interpretability
Creating highly interpretable AI models might sacrifice the overall performance and complexity of the system. Striking the right balance is crucial.
2. Scalability
As AI models grow in complexity and size, generating explanations for each decision becomes computationally intensive, requiring scalable solutions.
3. Human-Centric Explanations
AI explanations should be tailored to human understanding, avoiding technical jargon and complex mathematical terms.
FAQs About Explainable AI
How does Explainable AI differ from traditional machine learning?
Traditional machine learning models often lack transparency, making it challenging to understand how they arrive at specific decisions. Explainable AI, on the other hand, focuses on providing interpretable explanations for AI model outputs, making the decision-making process transparent and understandable.
What industries benefit most from Explainable AI?
Explainable AI has significant applications in industries like healthcare, finance, autonomous vehicles, and fraud detection, where transparent decision-making is critical for user trust and regulatory compliance.
Is Explainable AI applicable to all AI models?
Yes, Explainable AI techniques are applicable to a wide range of AI models, including neural networks, decision trees, and ensemble models.
Can Explainable AI help in identifying biased AI models?
Absolutely! Explainable AI is instrumental in identifying biases and discriminatory patterns within AI models, enabling organizations to address and eliminate them.
How does Explainable AI contribute to ethical AI practices?
Explainable AI fosters ethical AI practices by promoting transparency, accountability, and fairness in AI decision-making, ensuring that AI systems are used responsibly and without harm.
Are there any regulatory requirements for using Explainable AI in certain industries?
Yes, certain industries, such as healthcare and finance, are subject to regulatory requirements for transparent and interpretable AI models to ensure compliance and ethical practices.
Conclusion
In conclusion, Explainable AI stands at the forefront of the AI revolution, unveiling the secrets of machine learning and promoting transparent and interpretable AI models. By fostering trust, enabling ethical practices, and providing valuable insights, Explainable AI empowers businesses and society to harness the full potential of artificial intelligence. As we journey into a future where AI becomes an integral part of our daily lives, the understanding of AI's decision-making processes becomes paramount. With Explainable AI, we unlock the power of AI for the betterment of humanity, ensuring that AI remains a force for good in a world of endless possibilities.