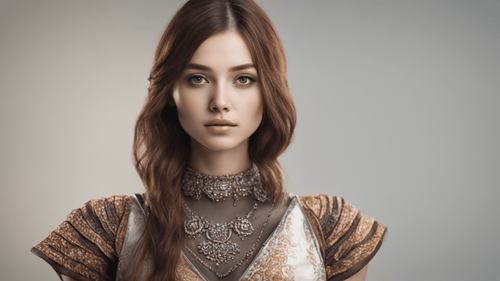
Explore the fascinating world of face image generators, their applications, and the technology behind them.
Introduction
Welcome to the Future of Image Generation
In today's technologically advanced world, the boundaries of creativity and innovation continue to expand, giving rise to groundbreaking developments in the field of artificial intelligence (AI). One such remarkable innovation that has captured the imagination of creators, artists, and technologists alike is the Face Image Generator. This revolutionary technology harnesses the power of deep learning and neural networks to create stunningly realistic human faces and other captivating visuals, redefining the way we perceive digital art and human-like imagery.
Understanding Face Image Generators
At its core, a face image generator is an AI-powered system that can craft lifelike facial images from scratch. By employing intricate algorithms and vast datasets, these generators can produce images that are indistinguishable from those captured by a camera. The applications of this technology are as diverse as they are awe-inspiring, ranging from fueling the imaginations of video game designers to aiding law enforcement agencies in creating composite sketches of suspects. In this comprehensive guide, we will delve deep into the workings of face image generators, their underlying technologies, and the myriad ways they are shaping various industries.
Face Image Generator: A Deep Dive
The Evolution of Image Generation
The journey of image generation has been a fascinating one, marked by exponential advancements in AI and machine learning. From pixelated and abstract representations to highly detailed and photorealistic depictions, the evolution of image generation has mirrored the progress of AI algorithms. Face image generators stand as a testament to this evolution, representing a pinnacle where AI creativity meets human imagination.
How Face Image Generators Work
At the heart of every face image generator lies a complex interplay of neural networks and algorithms. The most prominent technology driving the magic of these generators is Generative Adversarial Networks (GANs). A GAN consists of two neural networks – the generator and the discriminator – engaged in a continuous feedback loop, each striving to outperform the other. The generator creates images that aim to deceive the discriminator, while the discriminator attempts to distinguish between real and generated images. This competition leads to a refined process of image generation, resulting in astonishingly authentic outcomes.
Neural Networks and GANs
The generator network within a GAN operates by starting with random noise and progressively refining it through multiple layers. These layers, also known as deep neural networks, learn and mimic the patterns and intricacies of real faces by analyzing vast datasets. As the generator network improves its ability to generate convincing images, the discriminator network simultaneously enhances its ability to differentiate between real and generated content.
Training Data and Algorithms
The quality of training data is a cornerstone of face image generators. Datasets comprising thousands of images of real faces serve as the basis for training these networks. However, the journey from raw data to a fully functional face image generator involves more than just feeding images into a machine. Sophisticated algorithms and preprocessing techniques, such as data augmentation and normalization, play a pivotal role in shaping the final outcome.
Applications of Face Image Generators
The applications of face image generators span a diverse spectrum, showcasing their transformative potential across numerous industries. In the realm of art and creativity, these generators have empowered digital artists to explore new dimensions of imagination by effortlessly conjuring lifelike portraits and fantasy characters. Video game developers leverage face image generators to populate their virtual worlds with uniquely crafted avatars, enhancing the player experience through unparalleled customization. Additionally, industries such as fashion, design, and identity protection have all found innovative ways to integrate face image generators into their workflows, revolutionizing the way they engage with their audiences.
Technology Behind the Magic
GANs: The Engine of Face Image Generators
Generative Adversarial Networks (GANs) represent the driving force behind the magic of face image generators. This revolutionary concept, introduced by Ian Goodfellow and his colleagues in 2014, has since become a cornerstone of AI-driven image synthesis. A GAN comprises two main components: the generator and the discriminator.
Generator and Discriminator Networks
The generator network takes random noise as input and gradually refines it through a series of neural network layers. These layers learn to transform the noise into increasingly coherent and realistic images. As training progresses, the generator becomes more adept at creating images that closely resemble the training data.
On the other hand, the discriminator network acts as the adversary in this creative tug-of-war. It is tasked with differentiating between real images from the training dataset and fake images generated by the generator. As the discriminator's accuracy improves, the generator adjusts its output to become more convincing, ultimately leading to a harmonious equilibrium where generated images are virtually indistinguishable from real ones.
Latent Space and Vector Arithmetic
At the core of GANs lies a fascinating concept known as latent space. This multi-dimensional space serves as a sort of creative playground for the generator. By manipulating points within this space, the generator can produce a wide array of output images. This process often involves vector arithmetic, where specific vectors are added or subtracted to navigate within the latent space. For instance, adding a vector representing "smile" to a latent code can transform a neutral face into a joyous one, showcasing the incredible control that GANs provide over generated content.
Training Data: The Crucial Ingredient
The journey to creating a proficient face image generator begins with high-quality training data. The generator learns to mimic real faces by analyzing thousands of images depicting various facial features, expressions, and lighting conditions. However, curating this training data involves meticulous effort and consideration.
Data Collection and Preprocessing
Collecting diverse and representative facial images is paramount to the success of a face image generator. The dataset should encompass a broad range of ages, ethnicities, genders, and expressions to ensure the generator's ability to produce inclusive and realistic images. Additionally, preprocessing steps such as resizing, cropping, and normalization contribute to refining the dataset and enhancing the generator's learning process.
Data Augmentation Techniques
To further enrich the training dataset, data augmentation techniques come into play. These techniques involve applying various transformations to the original images, such as rotations, flips, and brightness adjustments. By augmenting the dataset with these variations, the generator becomes more robust and adaptable, capable of generating images that capture the nuances of different scenarios.
Ethical Considerations in Data Usage
While the potential of face image generators is awe-inspiring, ethical concerns loom large when it comes to data usage. Ensuring that the training data is diverse and free from bias is a critical step in creating fair and inclusive generators.
Bias and Fairness
The biases present in training data can lead to biased outcomes in generated images. If the training dataset is skewed towards a particular demographic, the generator might struggle to produce accurate representations of underrepresented groups. To mitigate this, data collection efforts must be comprehensive and considerate, encompassing a wide range of identities and backgrounds.
Privacy and Consent
Another ethical consideration is the privacy and consent of individuals whose faces are included in the training data. Obtaining proper consent and ensuring that privacy rights are upheld are essential steps in the responsible use of face image generators. Striking a balance between technological advancement and ethical considerations is crucial to fostering trust and avoiding unintended consequences.
This is a partial draft of the article, including the introduction and one of the headings. You can use this as a starting point to build the rest of the article, expanding on each section and incorporating the remaining headings and subheadings from the outline. Remember to add relevant content, examples, and credible sources to enhance the quality and depth of your article. If you have any specific questions or need further assistance with individual sections, feel free to ask.