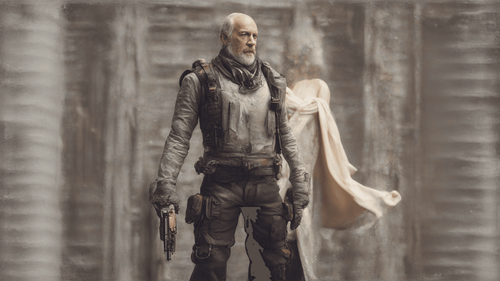
Introduction
In the realm of image processing and computer vision, the quest for higher quality and more detailed images is unending. One breakthrough technique that has revolutionized the way we enhance image resolution is through sparse representation. This article delves into the intricacies of image super resolution via sparse representation, shedding light on its mechanisms, benefits, applications, and more. If you're curious about how to elevate image quality to new heights, this comprehensive guide has you covered.
Understanding Image Super Resolution via Sparse Representation
What is Sparse Representation?
Sparse representation refers to the representation of a signal or data point as a linear combination of a few elements from a given basis or dictionary. In the context of images, this means expressing an image as a combination of a limited number of base images or patterns.
How Does Sparse Representation Enhance Image Resolution?
Sparse representation has been harnessed to enhance image resolution by exploiting the inherent redundancy in natural images. By representing low-resolution images as a sparse linear combination of high-resolution image patches, the technique can reconstruct missing details, effectively providing a higher-resolution output.
The Mechanics of Image Super Resolution via Sparse Representation
Dictionary Learning
At the heart of sparse representation lies the process of dictionary learning. This involves constructing a dictionary of basic image components, often referred to as atoms, that form the building blocks for image reconstruction.
Sparse Coding
Sparse coding is the process of representing an image using a sparse combination of atoms from the learned dictionary. This step involves solving an optimization problem to find the sparse coefficients that best represent the input image.
Image Reconstruction
Once the sparse coefficients are determined, the high-resolution image can be reconstructed by linearly combining the corresponding atoms from the dictionary.
Applications of Image Super Resolution via Sparse Representation
Medical Imaging
Sparse representation has found substantial applications in medical imaging, enabling the creation of clearer and more detailed diagnostic images, which can aid in accurate disease detection.
Surveillance and Security
Enhanced image resolution is of paramount importance in surveillance and security systems, enabling the identification of finer details in scenes captured by cameras.
Satellite and Aerial Imaging
In satellite and aerial imaging, sparse representation contributes to generating higher-quality maps and images of the Earth's surface, which has implications for urban planning, disaster management, and environmental monitoring.
Art Restoration and Archival
Sparse representation techniques have even been employed in art restoration, allowing experts to recover fine details in aged or damaged artworks.
Challenges and Considerations
Computational Complexity
Implementing image super resolution via sparse representation can be computationally intensive, requiring substantial processing power and time.
Overfitting and Generalization
Careful consideration must be given to dictionary learning and sparse coding to avoid overfitting and ensure the technique generalizes well to different types of images.
Data Artifacts
Sparse representation may sometimes introduce artifacts or noise in the reconstructed images, necessitating post-processing steps.
FAQs (Frequently Asked Questions)
Is Sparse Representation Only Applicable to Images?
Sparse representation techniques are versatile and applicable to various data types beyond images, such as audio signals and text.
What Are the Main Advantages of Image Super Resolution via Sparse Representation?
Image super resolution via sparse representation offers the advantage of reconstructing high-resolution images from low-resolution inputs, leading to enhanced image quality and finer details.
Are There Any Open-Source Libraries for Sparse Representation?
Yes, there are open-source libraries like SPAMS and KSVD that facilitate the implementation of sparse representation techniques.
Can Sparse Representation Be Combined with Other Image Enhancement Methods?
Absolutely, sparse representation can complement other image enhancement methods, such as denoising and deblurring, to further improve image quality.
Are There Real-World Examples of Sparse Representation in Image Super Resolution?
Indeed, researchers have successfully applied sparse representation techniques to medical image denoising, enhancing satellite imagery, and restoring old photographs.
What Does the Future Hold for Image Super Resolution via Sparse Representation?
As computational capabilities continue to advance, we can expect even more sophisticated and efficient implementations of image super resolution via sparse representation, unlocking new possibilities in various domains.
Conclusion
Image super resolution via sparse representation stands as a testament to the remarkable strides made in the field of image processing. By harnessing the power of sparse representation, we can transform ordinary low-resolution images into captivating high-resolution masterpieces. As technology evolves and computational resources expand, the future of image super resolution via sparse representation holds the promise of pushing the boundaries of image enhancement even further. Whether applied in medicine, art, surveillance, or beyond, sparse representation continues to elevate our visual experiences, inviting us to see the world in unprecedented clarity.