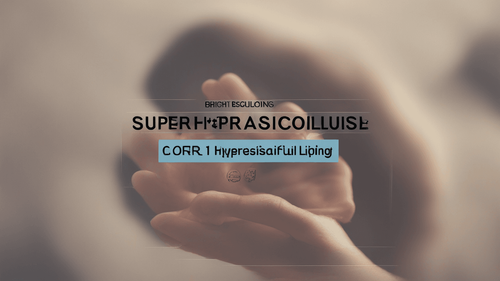
Introduction
In the ever-evolving realm of image processing, a revolutionary technology has emerged, reshaping our perception of visual content. Single Image Super Resolution (SISR), a cutting-edge technique, holds the key to elevating the quality of images to unprecedented levels. This article delves deep into the world of SISR, uncovering its mechanisms, applications, and transformative impact on various industries.
Single Image Super Resolution: Enhancing Visual Fidelity
Understanding the Essence of SISR
At its core, Single Image Super Resolution refers to the process of enhancing the resolution and quality of a single low-resolution image to produce a high-resolution counterpart. The goal is to extract finer details and improve overall clarity, all while retaining the authenticity of the original content. Through intricate algorithms and advanced neural networks, SISR algorithms work their magic, generating astonishingly enhanced images.
The Science Behind the Magic
SISR's success hinges on the utilization of convolutional neural networks (CNNs) and machine learning techniques. These networks, trained on vast datasets of high and low-resolution image pairs, learn to map low-frequency features from the low-res input to high-resolution space. As a result, SISR algorithms can predict and fill in the missing details, effectively upscaling the image.
Applications That Shine with SISR
The applications of SISR span a diverse range of fields, from photography and entertainment to medical imaging and satellite imagery. Digital Restoration: Preserving invaluable historical photographs or restoring old family pictures becomes a breeze with SISR. Art and Creativity: Artists can breathe new life into their works by upscaling and refining their digital creations. Medical Advancements: SISR aids in medical diagnosis by enhancing the clarity of intricate scans, enabling more accurate assessments.
Unveiling the Mechanics of SISR
Key Steps in SISR Process
- Image Preprocessing: The input image undergoes initial preparation to standardize formats and eliminate noise.
- Feature Extraction: Low-resolution image features are extracted to guide the enhancement process.
- Super-Resolution Mapping: Neural networks create a mapping between low and high-resolution features.
- Image Reconstruction: High-resolution features are combined and refined to reconstruct the final image.
Training SISR Models
The prowess of SISR hinges on robust training. Large datasets of paired low and high-resolution images are used to train CNNs. During training, the network learns to decipher intricate patterns and relationships, allowing it to generate high-resolution images from low-res inputs.
Debunking Common Queries about SISR
How Does SISR Differ from Traditional Upscaling?
SISR stands miles apart from traditional upscaling methods. Unlike simplistic algorithms that interpolate pixel values, SISR employs complex neural networks, allowing for the generation of genuine high-frequency features and finer details.
Is There a Limit to Upscaling with SISR?
While SISR performs wonders, it isn't magical. Enlarging an image beyond a certain point might lead to diminishing returns, as genuine details become scarce. However, the boundaries are continually pushed through innovative research.
Can SISR Alter the Original Content?
SISR is designed to enhance, not alter. It retains the essence of the original image, merely accentuating finer points and textures that might be imperceptible in the low-resolution version.
Is SISR Computationally Intensive?
Yes, SISR does demand computational resources, particularly for real-time applications. However, advancements in hardware and optimization techniques are steadily reducing this strain.
Does SISR Work Equally Well on All Image Types?
SISR's effectiveness can vary based on image content and quality. Images with intricate details and textures tend to benefit the most from SISR's magic.
Can SISR Be Applied to Videos?
Indeed, SISR's principles can be extended to video sequences, leading to the enhancement of video quality through frame-by-frame processing.
Embracing the Future: SISR's Evolution and Beyond
As technology gallops ahead, SISR continues to evolve. Researchers are exploring novel architectures, such as Generative Adversarial Networks (GANs), to push the boundaries of image enhancement. The fusion of SISR with other AI-driven processes opens doors to even more remarkable possibilities, from holography to 3D rendering.
Conclusion
In the dynamic landscape of image processing, Single Image Super Resolution stands as a testament to human ingenuity. Its ability to unravel hidden intricacies, breathe life into old memories, and elevate artistic expression is truly awe-inspiring. As SISR evolves, our visual experiences are destined to become more immersive, captivating, and astonishingly lifelike.