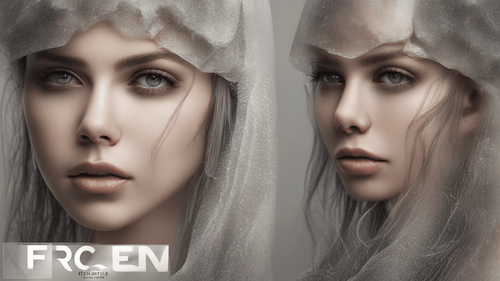
Introduction
In the realm of image processing and generation, Super-Resolution Generative Adversarial Networks (SRGAN) have emerged as a revolutionary technology. SRGANs offer a sophisticated approach to enhancing the visual quality of images, unlocking numerous applications across various industries. In this article, we'll delve into the intricacies of SRGANs, exploring their mechanisms, real-world applications, and the future prospects of image generation.
Understanding SRGANs
What are SRGANs?
SRGANs, short for Super-Resolution Generative Adversarial Networks, are a class of deep learning models designed to enhance the resolution and overall quality of images. Unlike traditional interpolation techniques, SRGANs leverage the power of adversarial training to generate high-resolution images that closely resemble their high-quality counterparts.
How do SRGANs Work?
SRGANs consist of two key components: a generator and a discriminator. The generator is responsible for upscaling low-resolution images, while the discriminator evaluates the generated images against real high-resolution images. Through an iterative process, the generator and discriminator engage in a tug-of-war, resulting in the generator producing images of remarkable visual fidelity.
The Role of Deep Convolutional Networks
Deep Convolutional Networks (DCNs) serve as the backbone of SRGAN architecture. These networks are adept at capturing intricate features and patterns within images, enabling SRGANs to generate high-resolution images with fine details.
Applications of SRGANs
Enhancing Visual Quality in Photography
SRGANs find extensive use in the field of photography, where they can transform low-resolution images into stunning high-resolution masterpieces. Photographers and artists can now salvage imperfect shots and turn them into works of art.
Medical Imaging Advancements
In the realm of medical imaging, SRGANs contribute significantly to enhancing the clarity of scans, allowing medical professionals to analyze images with greater precision. This breakthrough technology aids in accurate diagnoses and treatment planning.
Revolutionizing Satellite Imagery
SRGANs play a pivotal role in satellite imagery, where high-resolution visuals are crucial for various applications, including environmental monitoring, disaster management, and urban planning. SRGANs enable clearer and more detailed satellite imagery, empowering decision-makers with actionable insights.
Elevating Video Content Quality
Video content creators benefit from SRGANs by upscaling video frames, leading to improved video quality. Whether it's restoring old movies or enhancing real-time video streams, SRGANs offer a new dimension of visual fidelity.
The Future of Image Generation: What Lies Ahead
Advancements in Generative Models
As SRGANs continue to evolve, the field of generative models is poised for remarkable growth. Researchers are exploring novel architectures and techniques that promise even more realistic and higher-resolution image generation.
Cross-Domain Applications
The versatility of SRGANs extends beyond image enhancement. There's growing interest in applying SRGANs to cross-domain tasks, such as transforming sketches into realistic images or converting black-and-white photos into vibrant color renditions.
Ethical Considerations and Challenges
With great power comes great responsibility. As SRGANs become more sophisticated, ethical concerns surrounding image manipulation, privacy, and authenticity emerge. Striking a balance between technological advancement and ethical implications will be a critical aspect of SRGAN's journey.
FAQs (Frequently Asked Questions)
How is SRGAN different from traditional upscaling methods?
SRGANs differ significantly from traditional upscaling methods by leveraging deep learning and adversarial training. Traditional methods often result in blurry or unrealistic images, while SRGANs produce visually appealing and detailed high-resolution images.
Can SRGANs be used for video game graphics enhancement?
Absolutely! SRGANs can enhance the visual quality of video game graphics by upscaling textures and improving image details. This can lead to more immersive gaming experiences for players.
Are there any limitations to SRGAN technology?
While SRGANs offer remarkable results, they are not without limitations. Training SRGANs requires substantial computational resources, and there may be instances where certain image features are challenging to reproduce accurately.
How do SRGANs contribute to AI-generated art?
SRGANs play a pivotal role in AI-generated art by transforming low-resolution sketches or images into high-quality, visually appealing artworks. This allows artists and creators to explore new realms of creativity.
Are there any potential applications of SRGANs in autonomous vehicles?
Indeed, SRGANs could find applications in enhancing the quality of visual input for autonomous vehicles. Clearer and more detailed images can aid in object detection, improving the safety and reliability of self-driving systems.
What are the key research areas in SRGAN development?
Researchers are actively working on improving SRGANs' efficiency, training stability, and cross-domain adaptation. Exploring novel loss functions, network architectures, and training strategies are essential areas of focus.
Conclusion
In the rapidly evolving landscape of image generation, Super-Resolution Generative Adversarial Networks (SRGANs) stand as a testament to the incredible potential of deep learning. These remarkable models not only enhance visual quality but also open doors to a myriad of applications across diverse industries. As SRGANs continue to evolve, researchers and practitioners are on the brink of unlocking even more astonishing advancements in the world of image processing and generation.