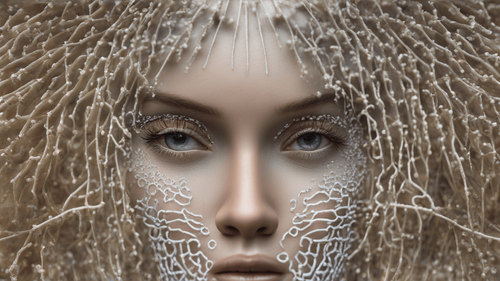
Introduction
In the ever-evolving landscape of artificial intelligence, the stable diffusion neural network has emerged as a remarkable innovation that holds the promise of revolutionizing various AI applications. This neural network variant combines stability and diffusion processes, resulting in enhanced performance and robustness. In this comprehensive guide, we will delve into the intricacies of the stable diffusion neural network, its applications, advantages, and potential future impact.
Stable Diffusion Neural Network: Unveiling the Concept
What is a Stable Diffusion Neural Network?
A stable diffusion neural network is a sophisticated computational model that integrates stability theory and diffusion processes within neural networks. It aims to enhance learning dynamics and improve the convergence rate of neural network training. By leveraging the principles of stability and diffusion, this network architecture introduces a novel approach to optimizing weight updates and activations.
How Does it Work?
The stable diffusion neural network operates by blending stability-driven updates with diffusion-based information propagation. This unique combination enables more efficient weight adjustments during backpropagation, leading to faster convergence and reduced training times. The diffusion process facilitates the smooth spread of information across layers, enabling better feature extraction and representation.
Key Components of Stable Diffusion Neural Networks
- Stability-driven Updates: The network's stability-driven updates ensure that weight adjustments are controlled and guided, preventing abrupt changes that could hinder convergence.
- Diffusion Information Flow: The diffusion process promotes the gradual dissemination of information, allowing each layer to contribute meaningfully to the overall learning process.
- Adaptive Learning Rates: Stable diffusion networks often incorporate adaptive learning rates for different layers, enhancing training efficiency and convergence.
Applications of Stable Diffusion Neural Networks
Natural Language Processing (NLP) Advancements
Stable diffusion neural networks have showcased remarkable potential in NLP tasks such as sentiment analysis, machine translation, and text generation. The integration of stability and diffusion enhances contextual understanding, leading to more accurate language processing.
Image Recognition and Computer Vision
In the realm of image recognition and computer vision, stable diffusion neural networks have demonstrated improved feature extraction capabilities. This enables them to excel in tasks like object detection, image classification, and facial recognition.
Enhancing Reinforcement Learning
Stable diffusion networks have the potential to refine reinforcement learning algorithms by providing stable and consistent updates to policy networks. This stability ensures smoother convergence in complex environments.
Time Series Analysis and Forecasting
With their efficient diffusion-based information propagation, stable diffusion networks are well-suited for time series analysis and forecasting. They can capture intricate temporal patterns and make accurate predictions in various domains.
Advantages and Innovations
Accelerated Training Convergence
The integration of stability and diffusion expedites training convergence by preventing weight updates that could disrupt the learning process. This leads to faster model training and reduced computational overhead.
Robust Feature Representation
Stable diffusion networks offer robust feature representation through the gradual accumulation of information. This results in more informative and discriminative features, enhancing the network's overall performance.
Improved Generalization
By maintaining stability during weight updates and information propagation, stable diffusion networks often exhibit improved generalization capabilities. This allows them to better adapt to new, unseen data.
Mitigating Vanishing and Exploding Gradients
The diffusion process in stable diffusion neural networks helps alleviate the issues of vanishing and exploding gradients, which are common challenges in deep learning. This contributes to more stable and effective training.
FAQs (Frequently Asked Questions)
Can stable diffusion neural networks be applied to recurrent architectures?
Yes, stable diffusion networks can be adapted to recurrent neural architectures. The diffusion process enhances the recurrent connections, leading to improved sequential pattern recognition.
Do stable diffusion networks require specialized hardware?
While stable diffusion neural networks can benefit from hardware acceleration, they are not solely reliant on specialized hardware. They can be trained efficiently on standard GPUs.
Are stable diffusion networks limited to supervised learning?
No, stable diffusion networks can be applied to both supervised and unsupervised learning tasks. Their stable updates and diffusion-driven information flow are advantageous in various learning paradigms.
Can stable diffusion networks be combined with other regularization techniques?
Indeed, stable diffusion networks can be combined with regularization techniques such as dropout and L1/L2 regularization. This combination can further enhance model stability and prevent overfitting.
Are there any real-world applications of stable diffusion networks?
Absolutely, stable diffusion networks have found applications in autonomous driving, medical image analysis, financial forecasting, and more. Their stability and efficiency make them versatile for diverse domains.
What is the future outlook for stable diffusion neural networks?
The future of stable diffusion networks is promising. As AI continues to advance, these networks could become a fundamental building block for faster and more reliable neural network training and deployment.
Conclusion
In the realm of artificial intelligence, the stable diffusion neural network stands as a testament to the innovation and progress the field continues to witness. By seamlessly integrating stability and diffusion, this neural network variant has unlocked new avenues for accelerating training convergence, enhancing feature representation, and overcoming challenges that have plagued traditional networks. As we look ahead, the potential applications and impact of stable diffusion networks are boundless, paving the way for more efficient and powerful AI systems that can shape the future across industries and domains.