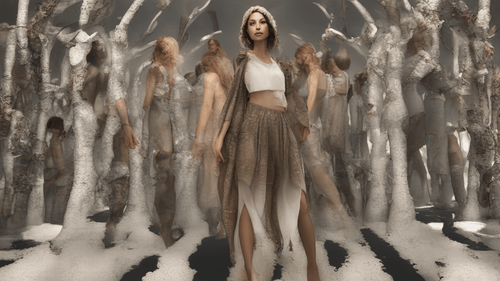
Introduction
In the realm of digital imagery, achieving high-resolution visuals is paramount for conveying detail and capturing the essence of a scene. Enter superresolution – an ingenious technique that takes image enhancement to the next level. In this comprehensive article, we'll delve into the captivating world of superresolution, understanding its mechanisms, exploring its various methodologies, and uncovering its potential applications across industries.
Superresolution: A Closer Look
Superresolution, often abbreviated as SR, is a groundbreaking image processing technique designed to enhance the resolution and quality of digital images. It employs sophisticated algorithms and data-driven approaches to upscale low-resolution images, unveiling intricate details that were previously invisible to the naked eye.
How Superresolution Works
Deep Learning and Neural Networks: The Powerhouses Behind SR
Superresolution harnesses the prowess of deep learning and neural networks to achieve its remarkable results. These AI-driven algorithms learn from vast datasets of high and low-resolution images, enabling them to make accurate predictions about missing image details during the upscaling process.
Single Image Superresolution vs. Multi-Image Superresolution
Superresolution techniques can be broadly categorized into single image and multi-image approaches. Single image SR involves enhancing a single low-resolution image, while multi-image SR leverages information from multiple images to reconstruct a high-resolution output.
Cutting-Edge Superresolution Techniques
Generative Adversarial Networks (GANs) for Superresolution
GANs have revolutionized the field of superresolution by pitting two neural networks against each other – a generator and a discriminator. The generator produces high-resolution images, while the discriminator evaluates their authenticity. This adversarial process leads to the creation of exceptionally realistic and detailed images.
Enhanced Super-Resolution Generative Adversarial Networks (ESRGAN)
ESRGAN takes GANs to the next level by incorporating a perceptual loss function and leveraging advanced architectural modifications. This results in sharper, more natural-looking images that exhibit a higher level of detail and realism.
Progressive Growing of GANs for Superresolution
The progressive growing technique involves training GANs on images of progressively increasing resolution. This approach ensures that the network gradually refines its ability to generate high-quality images, making it particularly effective for superresolution tasks.
Applications of Superresolution
Medical Imaging: Enhancing Diagnostics and Treatment
In the medical field, superresolution plays a pivotal role in enhancing the clarity of medical images such as X-rays, MRIs, and CT scans. This heightened visibility aids healthcare professionals in making accurate diagnoses and treatment decisions.
Satellite and Aerial Imagery: Sharpening Remote Sensing Data
Superresolution finds its place in satellite and aerial imagery, where it transforms low-resolution snapshots of Earth's surface into intricate and detailed maps. This has profound implications for environmental monitoring, urban planning, and disaster management.
Art Restoration: Breathing New Life into Masterpieces
Restoring centuries-old artworks often involves dealing with deteriorated images. Superresolution techniques breathe new life into these masterpieces by uncovering lost details and enhancing overall visual quality.
Frequently Asked Questions (FAQs)
How does superresolution differ from traditional upscaling methods?
Superresolution employs advanced AI algorithms to predict and generate high-resolution details, while traditional upscaling methods simply enlarge existing pixels, often resulting in blurry images.
Can superresolution be applied to video content?
Yes, superresolution techniques can also be extended to enhance the resolution of video content, providing smoother and more detailed playback.
Are there any limitations to superresolution?
While superresolution is remarkably powerful, it does have limitations. It relies on the quality of the training data and may struggle with generating entirely accurate details in complex scenes.
Is superresolution a resource-intensive process?
Yes, due to the complex nature of deep learning algorithms, superresolution can be computationally intensive. However, advancements in hardware and optimization techniques are making it more accessible.
What industries beyond those mentioned can benefit from superresolution?
Superresolution has applications in diverse industries, including astronomy, microscopy, and surveillance, where enhanced image quality is crucial for analysis and decision-making.
Can smartphones and cameras utilize superresolution?
Absolutely. In fact, many modern smartphones and cameras employ superresolution techniques to deliver sharper and more detailed photographs.
Conclusion
In the ever-evolving landscape of digital imagery, superresolution stands as a beacon of innovation, transforming ordinary visuals into extraordinary masterpieces. By harnessing the capabilities of artificial intelligence and advanced algorithms, superresolution has shattered the limitations of traditional upscaling methods, opening new vistas of possibilities across industries. As technology continues to march forward, we can only anticipate that superresolution will continue to redefine the boundaries of image enhancement, one pixel at a time.