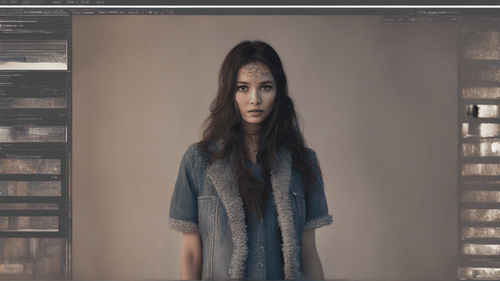
Introduction
In the dynamic world of image processing, the quest for higher resolution and enhanced visual quality has led to groundbreaking innovations. One such innovation is TensorFlow Super Resolution, a cutting-edge technique that promises to revolutionize image enhancement. In this comprehensive guide, we'll delve deep into the realm of TensorFlow Super Resolution, unraveling its intricacies, benefits, and real-world applications. Whether you're a seasoned developer or a curious enthusiast, join us on this journey to unlock the potential of visual excellence.
TensorFlow Super Resolution: Unveiling the Magic
Understanding TensorFlow Super Resolution
TensorFlow Super Resolution, often abbreviated as TFSR, is a state-of-the-art deep learning-based technique aimed at enhancing the resolution of images. It employs advanced neural network architectures to generate high-resolution versions of low-resolution input images, effectively breathing new life into visual content.
Key Components of TensorFlow Super Resolution
TFSR comprises several integral components working harmoniously to achieve impressive image enhancement:
-
Feature Extraction: The initial step involves extracting essential features from the low-resolution image, enabling the network to grasp intricate details.
-
Neural Network Architecture: TFSR employs convolutional neural networks (CNNs) or generative adversarial networks (GANs) to learn complex patterns and relationships within images.
-
Training Data: High-quality, high-resolution images are used to train the model, allowing it to learn from diverse visual examples.
The Inner Workings of TensorFlow Super Resolution
Training the Model
Training TFSR models involves presenting the neural network with pairs of low-resolution and high-resolution images. Through a process known as supervised learning, the model learns to map low-resolution inputs to their corresponding high-resolution outputs.
Loss Functions: Guiding the Learning Process
Loss functions play a pivotal role in fine-tuning the TFSR model. They quantify the disparity between generated high-resolution images and actual high-resolution targets, driving the model towards producing accurate results.
Inference: Transforming Low-Res to High-Res
During inference, the trained TFSR model takes center stage. It processes new, unseen low-resolution images and generates remarkably enhanced high-resolution versions, often surpassing the capabilities of traditional upscaling methods.
Applications of TensorFlow Super Resolution
Elevating Visual Media Productions
From film and television to online streaming platforms, TFSR holds the potential to elevate visual media to unprecedented heights. It can transform grainy frames into crystal-clear visuals, enhancing the overall viewing experience.
Medical Imaging Precision
Medical professionals can harness TFSR to enhance the clarity of medical images, aiding in accurate diagnostics and enabling more informed decision-making.
Art Restoration and Archiving
Preserving artistic heritage is another avenue where TFSR shines. By restoring aged artworks and historical photographs, this technique contributes to cultural conservation.
Implementing TensorFlow Super Resolution: A Step-by-Step Guide
Step 1: Data Collection and Preparation
Gathering a diverse dataset of high-quality images is paramount. Curating a mix of subjects, lighting conditions, and textures ensures robust model training.
Step 2: Model Selection and Customization
Choosing an appropriate neural network architecture sets the stage for success. Depending on the nature of your images, opt for CNNs or GANs, and fine-tune hyperparameters accordingly.
Step 3: Training and Validation
Divide the dataset into training and validation sets. Monitor the model's performance on the validation set to prevent overfitting and ensure generalization.
Step 4: Post-Processing and Refinement
Incorporate post-processing techniques such as denoising and sharpening to further enhance the output quality of the TFSR model.
Frequently Asked Questions (FAQs)
Is TensorFlow Super Resolution suitable for all types of images?
Absolutely! TensorFlow Super Resolution can be applied to various types of images, including photographs, medical scans, and even satellite imagery.
What hardware is recommended for training TFSR models?
Training TFSR models can be computationally intensive. High-performance GPUs are recommended for efficient and speedy model training.
Can TFSR-generated images be used for commercial purposes?
Yes, as long as you have the rights to the original low-resolution images and comply with any applicable licensing agreements.
How does TFSR compare to traditional upscaling methods?
Unlike conventional upscaling methods, TFSR generates high-resolution images with a deep understanding of visual features, resulting in superior quality and finer details.
Is fine-tuning a pre-trained TFSR model beneficial?
Indeed, fine-tuning a pre-trained model can expedite the training process and enhance the model's performance on specific image domains.
Are there any limitations to TensorFlow Super Resolution?
While TFSR is highly effective, it may face challenges with extremely low-resolution source images or scenes with complex textures.
Conclusion
TensorFlow Super Resolution stands as a testament to the remarkable strides made in image enhancement through deep learning. Its potential to transform the way we perceive and interact with visual content is undeniable. As you embark on your journey with TensorFlow Super Resolution, let your creativity and innovation shape the future of high-resolution imagery. Embrace the power of TFSR, and watch as your visual experiences reach new heights.